This question [1] has been ringing in my ears for more than a decade. Of course, we will never 'solve' misinformation. We will never live in a world of perfect information (thank goodness). Misinformation is intrinsic to human communication and manifests in many forms; it even exists in animal communication. Although imperfect, the question has been a guidepost for my research and teaching.
I have devoted a good portion of my professional career to at least slowing the spread of misinformation and mitigating its effects. I see our growing epistemic crisis as one of society's grand challenges, especially in light of new advances in generative AI [2]. Our success or failure will determine the success of other grand challenges like preventing the next pandemic, protecting our planet, and preserving democracy.
Originally trained as a theoretical biologist, I often think about the role that information, networks, and communication play in the evolution of life on earth. I have compared the dynamics of stomatal networks to decentralized computers [3], modeled the co-evolution of sociality and virulence [4, 5] and asked whether ignorance promotes democracy [6]. Now a card-carrying computational social scientist, I think about similar questions but in science and society: e.g., what are the mechanisms that drive consensus and counter-consensus in online social networks [7], what is the impact of recommender systems, and now AI, on the evolution of knowledge networks [8, 9], what is the role of perceived expertise in governing online misinformation [10], and what are the social and economic forces that slow and speed the evolution of science [11, 12, 13, 14]. I move back and forth between trying to understand what drives amplification of misinformation [15, 16] and investigating different intervention strategies to slow its spread [17, 18, 19].
The Centers for Disease Control did not overcount COVID deaths by 94%.
Yet the claim---that only 6\% of COVID deaths were due solely to COVID---went viral in August 2020. It started with a Facebook post by a chiropractor from Woodinville, Washington, not far from where I live. Within about a day, a version of the post was picked up by a QAnon influencer, tweeted and then retweeted by the U.S. President to his tens of millions of followers [3].
Misinformation can travel at woodinville-to-whitehouse speeds. It can influence perceptions and policy and impact people's health. It can start with strategic intent to downplay COVID deaths, but it can also start with honest, albeit biased, sensemaking, like it may have here. The chiropractor reported the numbers correctly but misinterpreted comorbidities. This occurs when a patient has two or more chronic conditions. It does not mean the patient died of multiple causes. It means the patient died of COVID but also had other existing, chronic conditions such as diabetes, heart disease, and hypertension.
Over the last decade, I have observed countless stories like this. They often involve numbers, 'experts', and a science or health-related issue. They are usually found on social media but not always. They begin innocently (although not always), and within a relatively short amount of time, go viral and become central to national conversations [8]. The stories coalesce social groups but also create social divisions, and because of the real-world consequences, provoke the following questions:
Are we seeing more or less of these stories? What are their origins and where online do they spread? What is their impact on human behavior and perception? How can we detect them earlier and slow their spread? And what are the agents of amplification?
Science is the greatest of human inventions. It has solved and continues to solve many of society's most pressing questions in human health, planetary wellness and economic viability. But one of Science's new challenges is the well being of Science itself. The reproducibility crisis, misaligned incentives and evaluations of scientists, diversity challenges, literature overload, publication bias, and out-of-date publishing models are just a few of the maladies of Science. Turning the microscope on Science itself - the Science of Science - is the focus of my research.
How is this different than the sociology and history of science, science policy or scholarly communication? Overlaps exist and methods are borrowed from these established disciplines, but the difference is the scale and kind of data, the methods and tools from data science, and the amalgam of these disciplines under one roof. It is difficult to understand literature overload or the reproducibility crisis if one does not examine, in parallel, what drives scientists to publish, what technologies they use to disseminate their findings, and their established norms for publishing.
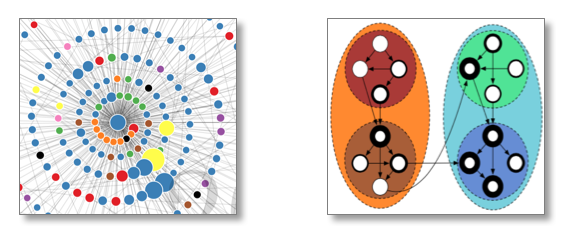
My research breaks into two categories: (1) knowledge science and (2) knowledge engineering.
(1) I study how scientists organize and communicate information. I look at gender bias in scholarly authorship. I develop methods for evaluating scientific impact. I look at the communication infrastructure and the social factors that lead to knowledge production in science.
(2) I develop tools and techniques for auto-categorizing and mining the literature, improving scholarly navigation (e.g., recommenders) and rethinking models of dissemination. My passion is to facilitate science and to keep this powerful machine running well. What I learn from knowledge science informs the recommender systems, search tools and mining techniques and vice versa.
Citation networks. As a biologist, I appreciate the utility of a good model system. Most of what we know about biology comes from a fruit fly, a worm and a small bacterium. A citation network is my model system for studying the flow of information. This model system is found, not within any one scholarly paper, but among the millions of scholarly papers that have been written over the last several centuries and the references that connect these papers. Initially, it was my interest in the history and sociology of science that attracted me to citation networks.
- How does the structure of science change over time?
- What paper or papers helped form or break apart existing fields?
- What is interdisciplinary science and what is its role in generating novel ideas?
Citation networks by themselves are interesting, but they also server as model systems for complex information networks of all kinds. One of the big challenges for understanding gene regulatory networks, ecosystem function or the evolution of virulence is to understand how the topology—the structure of these communication networks—affects function. The goal is the same with citation networks. The distinguishing quality is that a citation is relatively well defined, and the data is vast and easily accessible. The Eigenfactor Project provides some examples of the types of research that can be done with large, citation networks.
The scholarly graph is the keystone species in my research ecosystem. This consists of the millions of papers and billions of links that connect these papers. It includes the full text, figures, authors, and any other data associated with a scholarly article. It also includes connections to intellectual products on the web, in patents and in books. I have spent a good part of my academic career curating this scholarly graph using open data sources and content provided by publishers. It is from here that we can begin to ask questions about the origin and evolution of ideas and disciplines and how we can we use this to better facilitate science and innovation? I think of myself as a librarian with data science tools. I help scholars mine the literature in ways that go beyond a simple google scholar search.
The Scholarly Graph, In Aggregate. It is only in the last 5-10 years that researchers have been able to access and analyze the literature in aggregate. By aggregate, I mean the literature as a whole, not individual papers but millions of papers sewed together through citations, common language and across generations of scientists. Why not sooner? Publishers are reluctant to share their publications in bulk, and technologies for hosting and analyzing the literature at scale are just now becoming available. The arXiv, NIH mandate, and other Open Access initiatives are improving aggregate potential, but more needs to be done. There are many reasons to support Open Access, but for me, the reason is simple. There is a treasure trove that exists in the literature that can only be found through aggregate mining. No human has the capacity to read millions of publications and follow billions of links. Computers can. New advances in machine learning, natural language processing and network analytics are begging for a chance to go to work on scientific corpora. Humans can then interpret the nuggets mined.
What if you had the entire literature? What could you do differently in your field? What kinds of questions could you ask that you could not when only looking at individual papers? This is not a hypothetical any more. It is now possible and guides my research trajectory. It is a question I pose to collaborators and students. It often leads to interesting conversations and new questions. If you have an interesting answer, let's discuss and explore!
Science is a highly collaborative effort. It is no different for my research. I rely on my colleagues' expertise, students, university support, data providers and funders.
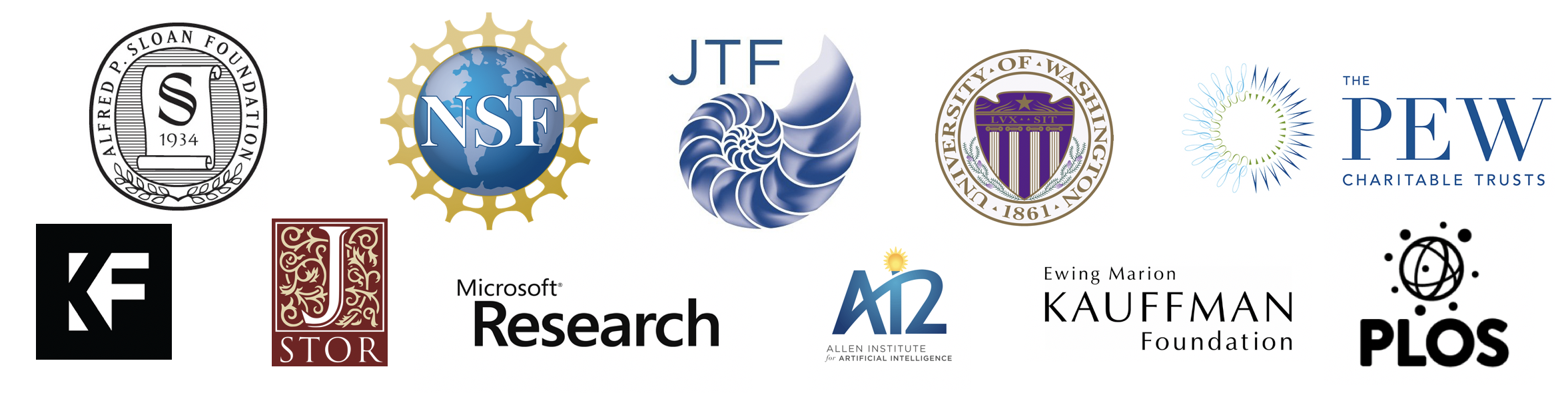